On My Summer Research
- Published by Infinite Wisdom
- Aug 20, 2024
- 4 min read
Emmanuel Paalam

While I hope everyone had a relaxing summer before their return—or admittance!—to UWF, I can’t say my summer was the same way. There was a lot on my plate to take on: as some of you may know, Infinite Wisdom has recently become its own, and we’ve recently separated from the Honors Council as its sister organization within the Honors program! While this decision permitted us greater creative freedoms, I’ve had to work on reorganizing a lot of stuff for this
site. From establishing a new set of guidelines to follow to developing a new staffhood, I was working hard to provide at least a partial foundation for Infinite Wisdom as this new season of its lifetime approached—and I hope you all remain able to interact with it!
But there’s another summer work experience of mine that I’d like to talk about today. Besides the obligation of overseeing the launching of a totally new chapter for this website, I also oversaw the beginning of something else that was new territory for me. I’m a Computer Science major, so it was definitely much more related to my major! But even then, machine learning model development, and learning about it as part of a research project I was part of for the summer, was an experience that required me to utilize my budding programming skills in a manner unfamiliar to me. It exposed me to things I’d never worked with before, and I had to do things I wasn’t used to—and, as hard as it was, it was a great opportunity for me to grow! My research, to keep things brief and skip the background introduction, aimed to develop a machine learning model to predict student dropout based on various factors, from academic performance to psychosocial and environmental attributes. The goal was to create a model that could identify students at risk of dropping out early, helping both students and educational institutions intervene before it's too late.
Hence, the benefits of my research! Simply put, I aimed to develop a machine learning model that can predict student dropout based on data on various student-related attributes, from straight-up academic performance to factors that may be more psychosocial, environmental, and overall contextual or non-academic. This model would then be able to take a student’s information and assess whether a student is at risk of dropout based on what is given (marking this a binary classification problem to solve). A model that can perform this task would be able to effectively tell students and their schools alike who’s in need of help. The only problem was, what kind of model would that be? I ventured to find out with the help of my lab.
But as cool as it all sounds, my research was still quite complicated. We used data from the Educational Longitudinal Study of 2002, provided by the National Center for Educational Statistics. This data tracked students from their sophomore year in high school until 2012, making it ideal for our study. My task involved testing various machine learning models, including logistic regression, Random Forest, and XGBoost, to determine the best approach. The work was challenging, with a steep learning curve, requiring me to preprocess data,
engineer model parameters, and seek help from various resources, including APIs, machine learning blogs, and ChatGPT.
It was a grueling summer, and there were times I questioned my decision to take on this project before its completion. However, the most rewarding part wasn't solving the research question—though I did, and XGBoost models proved to be great classifiers! It was when I presented my research at the Summer Symposium with a labmate who had worked on the same project. Creating the poster was a complicated process, and I almost thought we wouldn’t finish in time. But standing by our poster, presenting our work, and defending its importance was a proud moment. We received attention from fellow students, professors, and even industry professionals.
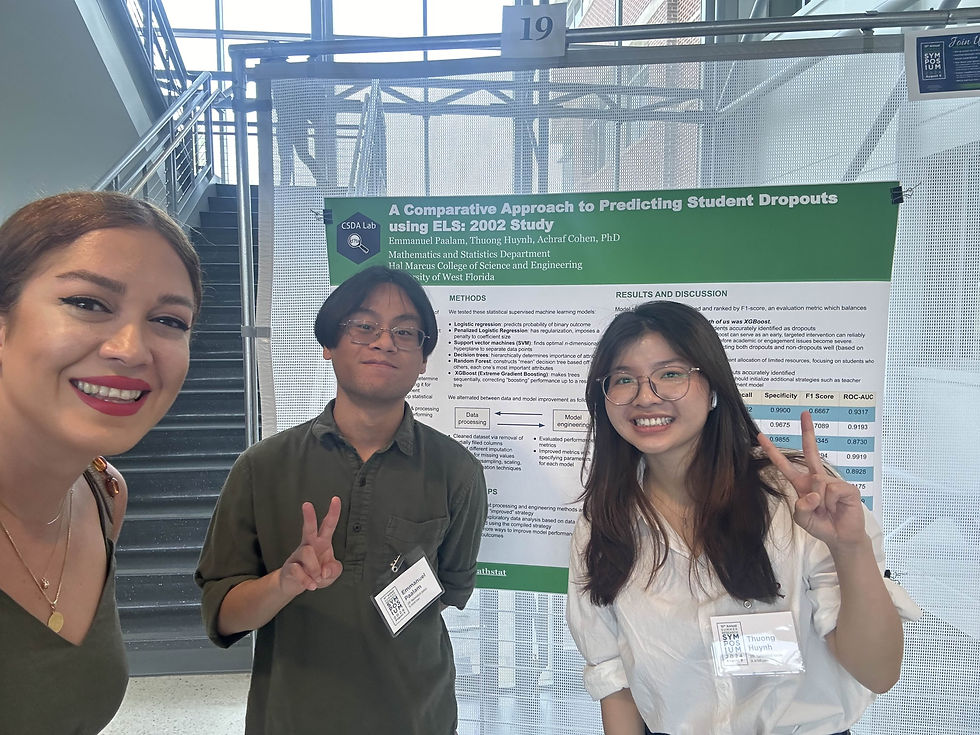
A photo of me, my labmate Anna, and Dr. Mahmoudian of the Movement Sciences and Health department.
This summer taught me a lot, even though it wasn’t always easy or enjoyable. My project will result in a paper, and I’ve already moved on to another, even deeper AI-focused project. This fall will be busy, but I’m prepared to tackle the challenges ahead. Like many of us in the Honors program, I’m driven by an end goal, even if it’s not always clear. I’m still figuring out the direction for Infinite Wisdom, but I’m committed to making progress despite my busy schedule.
I admit I’m still trying to figure out the end goal for Infinite Wisdom, even now working with my budding team on those guidelines and standards to set for ourselves. Where do I want to take this website for the term, as I slowly gather a team of fellow Honors students to help manage this site? Will I be able to do this for the term, seeing how busy I’ll be with classes and research as well? But for now, I’ll try not to get overwhelmed and remember what I learned over this summer, which I hope you guys take away too. Being resourceful is good! Use your resources, whether they be ChatGPT or actual tutoring, to help you learn. Work with your colleagues, in the class and out. But above all, don’t be afraid to accept that confusion and loss of understanding at times. That feeling just means you have expectations for yourself.
Comments